Personalization at scale is more than just a trending topic, it’s the future of ecommerce and digital marketing. Customer Data Platforms (CDPs) are a favored tool by marketers seeking to improve customer experience (CX) through relevant experiences and segmented communications. A CDP’s main strength is its data aggregation and unification capability - it breaks down data silos and can be used to build customer profiles across platforms and devices. CDPs are valuable, as they can help create segmented messaging and a more targeted approach to advertising, and their popularity amongst markers reflects a strong desire to strive for personalization.
At Particular Audience, we define meaningful personalization as the ability to serve intuitive predictions by matching real-time customer intent with relevant products. It is product data in fact, not customer data that bears the most fruit for prediction. The data is 1000x more useful than customer data alone.
In this post, learn what a CDP is, how it’s used, and how it sets the stage for the latest developments in advanced personalization technology, but is insufficient on its own.
So, what is a CDP?
A CDP is a database software that unifies a company’s first-party customer data from marketing and other channels, creating a ‘360-degree’ view of customers. Customer data can include personal attributes, behaviors, transactions and interactions with customer service. Before CDPs, data was stored in multiple locations such as CRMs (customer relationship management software, designed for sales departments and tracks sales-related data) and DMPs (data management platforms, designed for advertising departments and tracking ad-related data). CDPs consolidate customer data into one place and create some kind of persistent concept of identity around the customers. Some add communication capabilities on top, others simply feed into third party communication applications.
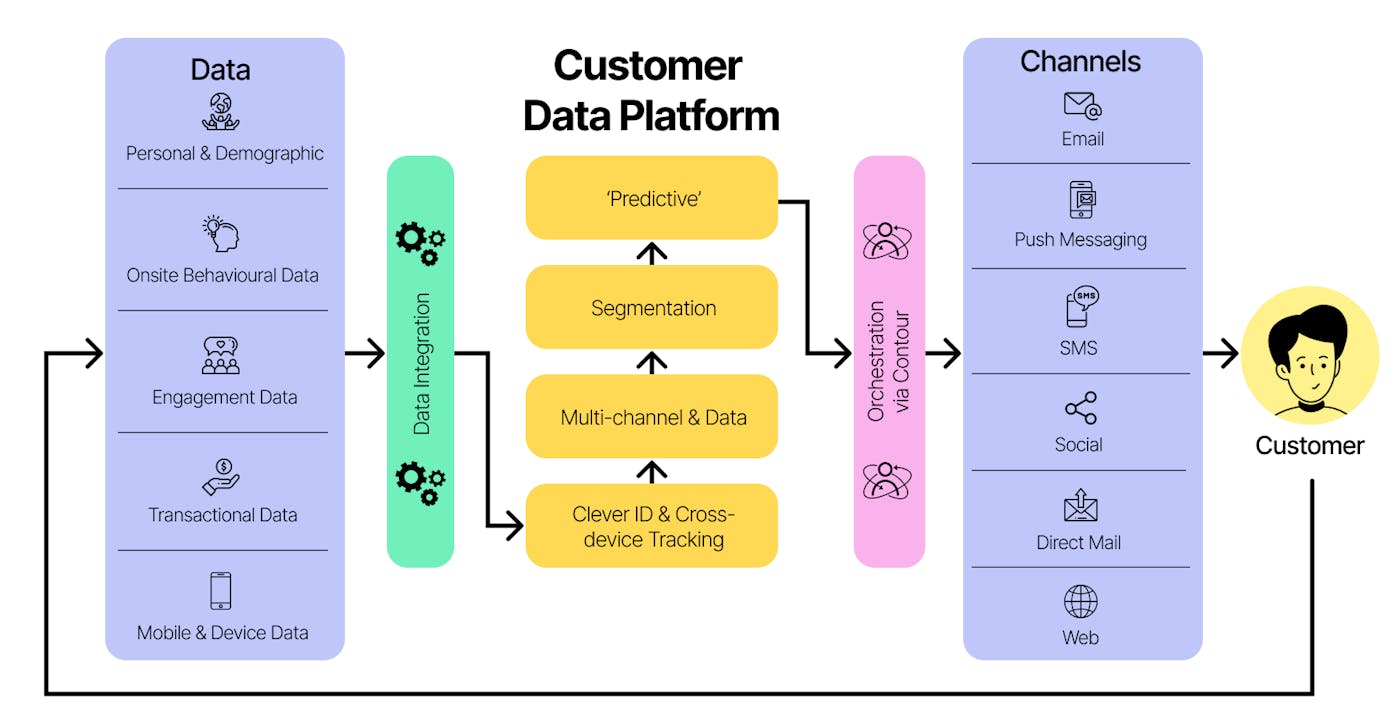
What data does a CDP use?
Most CDPs use first-party data collected directly from the customer, on the customer. Types of data include:
- Personal attributes - names, street addresses, email addresses, birthdays, education levels, etc.
- Behaviors - users' actions on a website or in a mobile app.
- Transactions - purchases, returns and other information garnered from POS and ecommerce systems.
- Customer service interactions - data from live chat sessions, session duration, number of sessions, NPS scores and other data collected by CRM systems.
CDPs also work with metrics from marketing campaigns (impressions, engagement, reach).
Because of a CDP's data collection capabilities, it’s a great starting point for personalization, in that contextual behaviors are logged and can become the subject of further personalization. A huge amount of aggregated data can be organized into a single customer view. However, the machine learning technology to leverage this vast data is not currently part of the package.
CDPs and ‘personalization’
Personalization is a hot topic in MarTech. Everyone wants it, but only a few companies are actually doing it well. As consumers demand exacting standards, it’s a no-brainer that marketers should meet expectations with seamless experiences across all platforms. You can’t provide personalized experiences if you don’t understand what your customers want or need, and you can’t do that without an accurate picture of their preferences — including the products and services they like, which channels they prefer and even how they like to be communicated with. Without this level of insight, you can only guess what your customers want from you, and run the risk of annoying them with irrelevant messages.
CDPs are a useful tool for segmenting customers according to their data and behavior, and have helped marketers create better and more consistent omni-channel experiences.
Currently, the process of using CDPs for ‘personalization’ looks like this:
- Develop customer intelligence at an individual level.
- Combine insights from customers’ experiences across multiple channels.
- Turn these insights into standardized data accessible to an entire tech stack.
- Create a closely-targeted, multi-channel experience in real-time.
CDPs increase efficiency, provide easier data access and help marketers better understand what customers want, need and expect. However, CDPs are a store of information, and there is no such thing as a ‘golden record’ for each customer. Limited, inaccurate, or incomplete customer information is an obstacle to personalization, and with privacy laws, opt-ins and the demise of third party cookies, marketers will have even less access to certain types of data going forward.
Given all this, marketers should ask themselves, is a CDP really everything I need?
Going beyond a CDP
There’s no doubt that CDPs are useful. They help organize data more effectively, allow marketers to recognize customers across different devices, and provide insight into what customers are doing in an app versus what they're doing on a webpage, as well as helping marketers segment messaging based on the customer data available.
An ever-growing pool of first party data and cross channel interactions is nice to have, but it’s even more valuable to be able to use data to deliver relevant personalized experiences and accurate predictions. While CDPs can make your communications and your messages relevant, they're not especially good at helping you predict future topics, items or trends relevant to your audience.
Why would you want to predict? Because meaningful personalization is good prediction.
Personalization stems from good prediction
In order to predict, you need a really robust data set. Consider the customer data you’re able to store in a CDP. You might know how a customer interacts across different devices and channels, their demographic information and what they’ve bought in the past, but this is not robust data - it represents a tiny fraction of a percent of the data points that would be available on any given person and how they spend their time online.
Think about how long you spend on a website and how much information you willingly give. Does this fraction of data shared capture the essence of who you are or what you want? Often not. For this reason, customers cannot be commoditized. Every single person is completely different. Their context and their intent is constantly changing. They might have bought an item for themselves from a site a few weeks ago, but today they could be shopping for their great aunt, or their brother’s newborn baby.
It’s also important to understand that customers are not the same as their demographic. What do we mean by this?
Consider Ozzy Osbourne and Prince Charles - two people with the same demographic data on paper. Same age, both are wealthy men, and both live in castles in England. And yet, you’d imagine their shopping carts look very different! With this in mind, trying to serve Ozzy predictions based on Prince Charles’ purchasing behavior doesn't make any sense. On the other hand, if you knew the items that Ozzy had recently looked at, and you knew what else customers who viewed those same items went on to click on or buy, you could serve Ozzy much better predictions based on this knowledge.
For exactly this reason, it is product data, not customer data, that is most effective for prediction in ecommerce.
Product data is valuable because you have:
- 100% of your item data.
- Every single item interaction.
- Every item purchased.
- Every search.
- Every item image.
- All of your product description data.
When you are able to understand the relationships between items (which items are similar to one another, which items go well together, which items are complementary to one another), then you can make predictions based on the in-moment intent of a customer, rather than demographic data or information about what a customer bought six months ago.
Advanced personalization platforms like Particular Audience use machine learning tech including computer vision to serve visually similar recommendations, as well as collaborative filtering tech to harness the wisdom of the crowd. By learning from the shopping journeys of hundreds of thousands customers, Particular Audience can better predict what customers want - if 100,000 people have followed a similar browsing journey, based on what they did next collaborative filtering can shortcut a customer’s path to the same outcome.
In short
CDPs have proved to be crucial tools for marketers looking to improve CX through personalized communications and omnichannel experiences, but the future of meaningful personalization is prediction, and product data is far more reliable than customer data for making predictions based on current intent.