A good idea means a bird’s eye view of the idea maze, understanding all the permutations of the idea and the branching of the decision tree, gaming things out to the end of each scenario. Anyone can point out the entrance to the maze, but few can think through all the branches. If you can verbally and then graphically diagram a complex decision tree with many alternatives, explaining why your particular plan to navigate the maze is superior to the ten past companies that fell into pits and twenty current competitors lost in the maze, you'll have gone a long way to proving that you actually have a good idea that others did not and do not have.
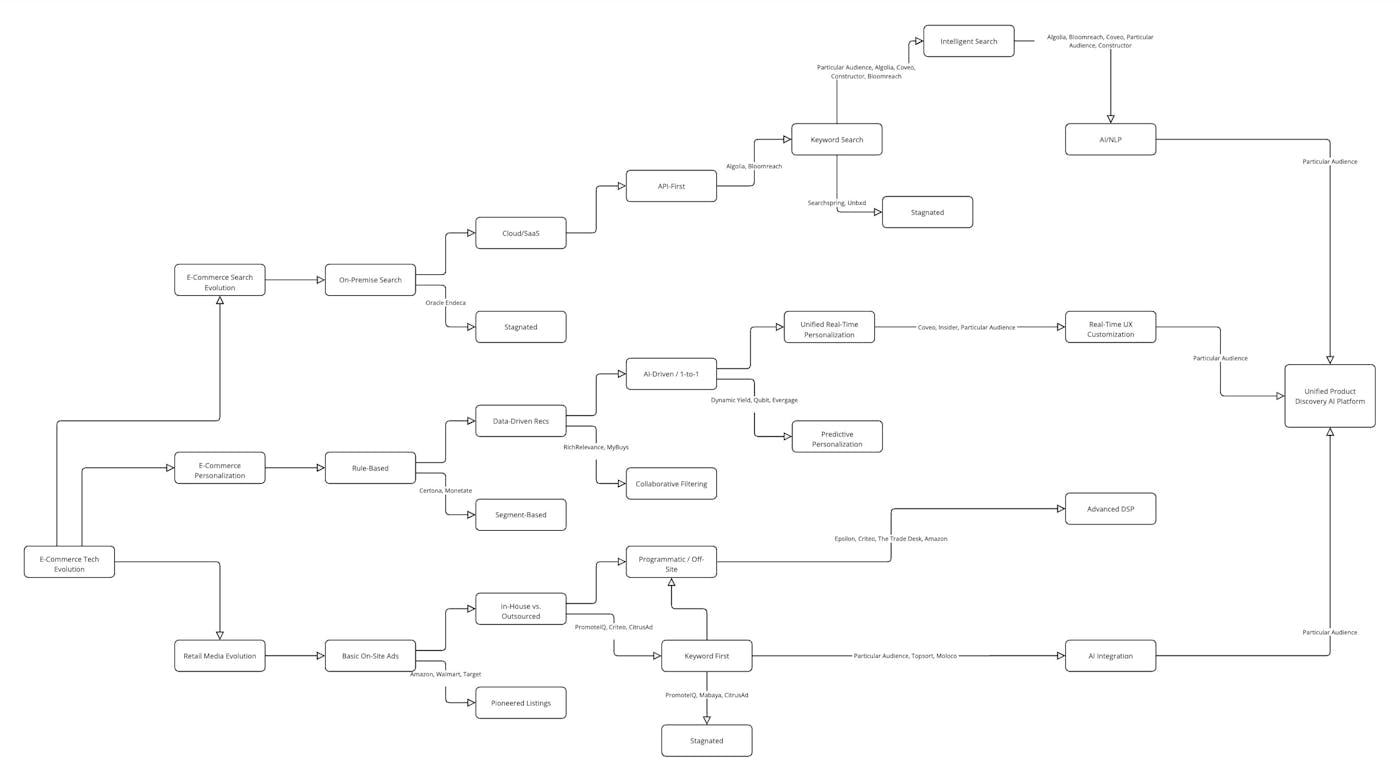
Evolution of Retail Media – An Idea Maze Map
E‑commerce technology has transformed dramatically over the past two decades. Below is a graphical decision tree outlining key branching points in the evolution of site search, personalization, and retail media. We highlight major technology shifts, vendor strategies, successes/missteps, and future directions. Particular Audience (PA) is woven throughout as a case study in unified, AI-driven innovation across search, personalization, and retail media.
Key Branching Points in the Evolution
- On-Premise vs Cloud Search: Early 2000s e-commerce search relied on on-premise engines (e.g. Oracle Endeca) installed with the e-commerce platform. The 2010s saw a pivot to cloud-based, API-first search services (e.g. Algolia, Bloomreach, Coveo) for faster innovation and scalability (Endeca migration - why now, why Algolia - Algolia Blog | Algolia) (Algolia Launches Algolia Recommend — A New API-First). Vendors who failed to embrace cloud delivery fell behind as SaaS eliminated burdens of upgrades and infrastructure (Endeca migration - why now, why Algolia - Algolia Blog | Algolia).
- Rule-Based vs AI-Driven Personalization: Initial personalization was rules-based (manual if/then segments, basic recommendations). Over time, machine learning and AI took over, enabling dynamic, hyper-personalized experiences at scale (What is Rule-Based Personalization? | Sitecore) (AI-powered personalization provider Qubit gets acquired by Coveo | VentureBeat). Companies that stuck to static rules saw engagement plateaus, whereas AI leaders (RichRelevance/Algonomy, Dynamic Yield, Qubit, etc.) delivered more relevant, real-time content and offers.
- Monolithic vs API-First Architecture: Earlier solutions were bundled into monolithic e-commerce suites, limiting flexibility. Newer “headless” or API-first models let retailers plug in best-of-breed search or personalization via simple APIs (Algolia’s search, Algolia Recommend with just “six lines of code” (Algolia Launches Algolia Recommend — A New API-First)). An API-first tech stack became the foundation for differentiated experiences in modern commerce (Algolia Launches Algolia Recommend — A New API-First).
- Manual Ad Sales vs Programmatic Retail Media: Retail media started with direct-sold ads on retailer sites (sponsored products banners managed manually). The late 2010s and 2020s brought programmatic retail media – automated auctions and off-site ad reach. Retailers evolved from basic on-site ads to sophisticated networks that include real-time bidding and off-site inventory, tapping DSPs like The Trade Desk and platforms like Criteo. Off-site programmatic retail media spend in the U.S. jumped from $7.5B in 2023 to $20B in 2024 (Retail media’s rise increasingly reliant on offsite programmatic media, report finds | Marketing Dive), highlighting this rapid shift.
- Third-Party Data vs Privacy-First Strategies: As third-party cookies wane, retailers’ first-party data became gold. The retail media boom is partly an answer to the death of third-party tracking – brands are reallocating ad budgets to privacy-safe, first-party data channels (Swiftly | Retail Media & Tech Insights | The Evolution of Retail Media). Future personalization will rely on consented data, CDPs, and zero PII techniques (as Particular Audience does with segment building without personal identifiers (Particular Audience Announces Largest Ever Product Release—Reinforcing Market Leadership in Advanced AI-Powered Retail Media, Search & Personalization | Business Wire)).
- Standalone vs Unified Platforms: Retailers historically used separate engines for site search, recommender systems, and advertising. A new approach is emerging: unified discovery platforms that combine organic search, personalized recommendations, and sponsored placements. Particular Audience exemplifies this by powering “both organic and sponsored personalized product discovery” in one AI-driven platform (Particular Audience Announces Largest Ever Product Release—Reinforcing Market Leadership in Advanced AI-Powered Retail Media, Search & Personalization | Business Wire), whereas legacy vendors offered these capabilities in silos.
Each branch above marks a critical decision point where vendors and retailers chose different paths – some adapting and thriving, others stagnating or failing. Below, we map these evolutions with vendor examples and outcomes.
Evolution of E‑Commerce Search
Early Era – On-Premise, Keyword-Centric Search (2000s): E-commerce pioneers integrated search into their platforms or via enterprise search tools. Oracle Endeca, once the gold standard, provided on-premise search and navigation for many top retailers (Endeca migration - why now, why Algolia - Algolia Blog | Algolia). This era relied on keyword matching and manually tuned relevance rules. Over time, these on-prem systems grew stale – for instance, Endeca went years without major updates under Oracle, effectively reaching “end of life” (Endeca migration - why now, why Algolia - Algolia Blog | Algolia). Vendors who failed to modernize (e.g. Endeca, Microsoft FAST for e-commerce) saw customers migrate away as their “legacy, unoptimized” search platforms started hurting business agility (Endeca migration - why now, why Algolia - Algolia Blog | Algolia). A key misstep was underestimating the rise of cloud and AI – leaving a gap that new players rushed to fill.
Branch – Shift to Cloud & API-First (2010s): Around 2010–2015, retailers faced the choice: stick with on-prem search or adopt cloud Search-as-a-Service. Many chose cloud. Algolia (founded 2012) championed a purely cloud, API-first search model: instead of heavy installations, developers could integrate lightning-fast search via API in days. This brought real-time indexing and instant search-as-you-type experiences unattainable in older systems. Algolia’s API-first approach is credited as “well suited to enable the future of commerce” (Algolia Launches Algolia Recommend — A New API-First). Similarly, Bloomreach launched a SaaS search and merchandising solution, later expanding into a full commerce experience cloud. Coveo, originally an enterprise search firm, rebuilt as a multi-tenant cloud platform and invested in AI relevance. The success of these cloud-native search providers is evident: they offered frequent improvements, scalability, and easy integration, which legacy on-prem vendors couldn’t match (Endeca migration - why now, why Algolia - Algolia Blog | Algolia). For example, Algolia guarantees 99.999% uptime across global data centers (Endeca migration - why now, why Algolia - Algolia Blog | Algolia), a reliability level hard to achieve in self-hosted systems. Vendors like Algolia and Bloomreach also embraced open APIs and headless commerce trends, making themselves compatible with any front-end.
In Parallel – Open Source & Cloud Hybrids: Some retailers experimented with open-source search (Apache Solr, Elasticsearch) as an alternative to licensed on-prem tools. Elastic provided cloud-managed versions as well. This allowed more control but required in-house expertise. The broad trend, however, was toward managed cloud solutions to reduce maintenance burden.
Branch – Intelligent, AI-Powered Search (late 2010s–2020s): As data and AI capabilities grew, search evolved beyond simple keyword matching. Leading platforms incorporated AI algorithms for semantic understanding and personalization. For instance, Coveo added machine learning models to detect shopper intent and context, enabling personalized search results for each user (AI-powered personalization provider Qubit gets acquired by Coveo | VentureBeat). Bloomreach integrated AI to optimize search and product ranking, including NLP to interpret queries. Google itself re-entered the space with Google Retail Search (a cloud API leveraging Google’s AI to improve on-site search relevance). The latest wave includes vector search and NLP models (like BERT/transformers) to grasp natural language queries and long-tail intent. This branch rewarded those who invested in AI R&D. Particular Audience pushed this further with its Adaptive Transformer Search – an AI search model that predicts shopper intent even without explicit keywords (Particular Audience Announces Largest Ever Product Release—Reinforcing Market Leadership in Advanced AI-Powered Retail Media, Search & Personalization | Business Wire). By moving beyond keyword-first search, PA and similar AI-first engines capture revenue from shoppers who browse or use natural language (traditionally missed by exact-match systems) (Particular Audience Announces Largest Ever Product Release—Reinforcing Market Leadership in Advanced AI-Powered Retail Media, Search & Personalization | Business Wire). The payoff of AI-driven search is higher relevance and conversion: for example, PA reported that replacing legacy “keyword-first” search with its transformer model doubled sponsored product fill-rates in one week (Particular Audience Announces Largest Ever Product Release—Reinforcing Market Leadership in Advanced AI-Powered Retail Media, Search & Personalization | Business Wire) (Particular Audience Announces Largest Ever Product Release—Reinforcing Market Leadership in Advanced AI-Powered Retail Media, Search & Personalization | Business Wire).
Outcome: Cloud and AI-driven search has largely won out. On-premise, purely keyword-based search is now considered obsolete for competitive retailers. A strategic success story here is Algolia’s rise from a niche API tool to a mainstream choice for countless websites by betting on cloud + speed + developer experience. In contrast, a misstep example is Google’s early Commerce Search product (launched 2009) which was discontinued by 2012 due to lack of flexibility – Google pivoted and later returned with a more AI-powered offering after seeing cloud rivals succeed. Oracle’s Endeca, as noted, stagnated and lost relevance (many clients migrated off by late 2010s). The decision tree branch of Cloud/API-first vs. Legacy On-Prem clearly saw the former thrive.
Notably, Particular Audience’s approach to search sits at the cutting edge of this evolution: it is cloud-native and AI-native. PA did not even offer a keyword-based solution – it jumped straight to transformer-based semantic search, essentially skipping a generation of technology. This positions it strongly against other search vendors by capturing subtle user intents and enabling retailers to unify product discovery across search and browse in an intelligent way.
Evolution of Personalization
Early Personalization – Manual Rules & Segmentation: In the 2000s, “personalization” in e-commerce was rudimentary. Retailers implemented rule-based campaigns – e.g. “If customer is in segment X, show banner Y,” or “Recommend top sellers in category”. These rules were often hard-coded or configured by marketers. They provided some uplift but were labor-intensive and not truly individualized. Emails would insert a first name or show items from a browsed category (basic targeting). This rule-based personalization relies on predefined logic and segments (What is Rule-Based Personalization? | Sitecore). It can’t adapt to complex patterns in real-time and quickly hits a ceiling in effectiveness, delivering generic, one-size-fits-all experiences that today’s customers ignore (What is Rule-Based Personalization? | Sitecore) (What is Rule-Based Personalization? | Sitecore). Early personalization vendors (e.g. Certona, Monetate) started with these approaches, and some e-commerce platforms offered built-in rule engines. The limitation became clear as online catalogs grew and consumer expectations rose.
Branch – Data-Driven Recommendations (late 2000s): A significant branching point was the adoption of algorithmic recommendations inspired by the likes of Amazon’s “Customers who bought X also bought Y.” Companies like RichRelevance (founded 2006) and MyBuys introduced collaborative filtering and analytics to personalization. Instead of static rules, these systems analyzed purchase history and product relationships to suggest relevant items (e.g. “frequently bought together” models). This was an early form of AI in retail, though often using simpler algorithms (matrix factorization, etc.). Retailers that embraced these data-driven rec engines saw higher average order values and engagement than those sticking purely to manual cross-sells. By 2010, recommendation widgets became common on product pages and cart pages, marking a success for this branch – though it mostly focused on product recommendations rather than full experience personalization.
Branch – AI-Driven, Individual Personalization (2010s): The next branch was a move from segment-level or aggregate patterns to truly 1-to-1 personalization using machine learning. Advances in AI enabled analyzing each shopper’s behavior in real time and predicting the best content or product for that specific person at that moment. Vendors like Dynamic Yield and Qubit (early 2010s) built ML-driven personalization platforms that could automatically test and tailor homepage banners, sort order, offers, etc. They moved beyond “people like you” recommendations to predictive personalization (e.g. predicting churn and offering a discount proactively, or re-ranking a category page differently for each user). Qubit in particular developed deep learning models for e-commerce and emphasized personalization for merchandising teams; it was later acquired by Coveo to infuse AI personalization into a broader relevance platform (AI-powered personalization provider Qubit gets acquired by Coveo | VentureBeat) (AI-powered personalization provider Qubit gets acquired by Coveo | VentureBeat). As Louis Têtu (Coveo’s CEO) noted at that acquisition, enterprises were turning to AI-driven solutions to deliver “highly relevant… recommendations to their customers at scale” (AI-powered personalization provider Qubit gets acquired by Coveo | VentureBeat). This wave saw successes like Dynamic Yield (acquired by McDonald’s in 2019 for its AI tech, later by Mastercard) and RichRelevance merging with personalization-focused fund (becoming Algonomy) to double-down on AI. On the other hand, vendors who remained largely rule-based started consolidating or fading – e.g. Certona, a rule/heuristic-based pioneer, got acquired and absorbed into larger platforms by 2019, as it struggled to compete with nimbler AI firms.
Integration of Personalization & Data Platforms (late 2010s): Another crucial development was integrating personalization engines with customer data platforms (CDPs) and broader marketing automation. Personalization was no longer confined to product recs on-site; it extended to email, mobile apps, and even in-store. For example, Bloomreach’s acquisition of Exponea (2021) combined a CDP with Bloomreach’s search and content engine, creating a unified customer and product data platform for commerce (Bloomreach Acquires Exponea and New Investment) (Bloomreach Acquires Exponea and New Investment). This gave marketers a 360° view to personalize across channels (web, email, SMS) using one system. Retailers that invested in omnichannel personalization (combining online behavior with in-store purchases via loyalty data) gained an edge in consistency. The trend underscores a success: Bloomreach became a leader by sitting “at the intersection of marketing, commerce, and AI” with an integrated suite (Bloomreach Acquires Exponea and New Investment). In contrast, some standalone personalization startups that didn’t expand beyond a point solution struggled; many were acquired or pivoted as features (e.g., Barilliance or Evergage, which Salesforce acquired to embed into its Marketing Cloud).
Hyper-Personalization & Real-Time AI (2020s): Today, the bar is set at hyper-personalization – using AI to tailor every aspect of the user journey in real time. This includes search results personalized to a user’s intent, dynamic pricing or offers, and content (images, copy) that adapt to each user. Particular Audience is a prime example of this modern branch: it treats personalization not as a separate module but as an AI layer underpinning search and retail media. PA’s platform “predicts intent before the customer even expresses it” (Particular Audience Announces Largest Ever Product Release—Reinforcing Market Leadership in Advanced AI-Powered Retail Media, Search & Personalization | Business Wire), meaning it can surface relevant products or ads proactively. By fusing personalization with search and ads, PA aims for a seamless experience where every touchpoint is tailored (if user A and user B search the same term, they might see different results or sponsored products based on their behavior). This is a differentiator versus competitors who might bolt on personalization as an afterthought. The results of hyper-personalization speak for themselves – retailers using AI personalization report significant conversion lifts. For instance, PA’s AI-powered recommendations and ads yield 1.1% click-through rates vs ~0.39% industry average (a 182% improvement) (Advanced Retail Media Technology). Such numbers illustrate why AI-driven personalization is now “mission-critical” and not just nice-to-have.
Outcome: The evolution of personalization shows a clear winner: AI-driven, real-time personalization is now the expected standard. Consumers respond to brands that remember their preferences and anticipate their needs. A strategic success story is Coveo’s transformation – originally search-focused, it recognized the need to incorporate personalization and acquired Qubit to deliver “personalized search, product recommendations... and optimization” in one platform (AI-powered personalization provider Qubit gets acquired by Coveo | VentureBeat). On the flip side, a notable misstep was any retailer who thought simple segmentation was enough – many saw engagement drop and eventually had to invest in AI personalization or lose customers to those who did. Even a tech giant like Netflix famously invested in a recommendation algorithm competition (the Netflix Prize) back in 2009 to improve suggestions – underlining how critical personalization had become.
Particular Audience’s role in personalization is particularly noteworthy: it represents the next phase where personalization is not a separate silo, but interwoven with discovery and monetization. By ensuring every ad or product shown is “hyper-personalized in real time” (Particular Audience Announces Largest Ever Product Release—Reinforcing Market Leadership in Advanced AI-Powered Retail Media, Search & Personalization | Business Wire), PA is pushing the envelope on what an e-commerce experience can be. This unified approach sets it apart from vendors that might excel in one area (say, pure recommendations) but not unify all channels. In the decision tree, PA chose all the forward-looking branches – AI over rules, real-time over batch, unified platform over point solution – making it an example of a company building on the lessons of the past to define the future.
Rise of Retail Media Networks
Origins – On-Site Retail Media (2010s): The concept of retail media — retailers selling ad placements on their own digital properties — took off with Amazon’s success. In the early 2010s, Amazon realized the value of its site traffic and launched an internal ad platform (sponsored product listings, banners on Amazon.com). By around 2012, Amazon had essentially created the first modern retail media network (Swiftly | Retail Media & Tech Insights | The Evolution of Retail Media), turning the point of purchase into advertising real estate. This opened a new revenue stream (today Amazon’s ad revenue is tens of billions). Other large retailers followed: Walmart, Target, Kroger, and others started to monetize their sites and apps with vendor-funded ads. Initially, many of these programs were rudimentary – e.g. static sponsored product slots or search keyword bidding systems. Criteo, an ad tech firm known for retargeting, began offering retailers technology to run their own site ads and use Criteo’s network of brands. PromoteIQ (founded as Spotfront) emerged as a vendor providing a turnkey on-site sponsored product platform, which Microsoft later acquired to pair with Bing ads. CitrusAd (founded 2017) offered “retail monetization in a box,” a self-serve system integrated into retailer websites (Peeling Back the Power of Retail Media: Q&A with CitrusAd | Tinuiti). These vendors positioned themselves to help mid-tier retailers launch an “Amazon-like” ads business quickly. Those who adopted early (e.g. Home Depot with PromoteIQ, some grocers with CitrusAd) gained incremental revenue with minimal investment – a success for both the retailer and the vendor. Meanwhile, retailers that ignored this trend left money on the table (essentially giving brands no choice but to spend on Amazon or Google to reach the same customers).
Branch – In-House vs Outsourced Platforms: A strategic branching happened as retail media matured: should a retailer build its own ad tech (like Amazon, Walmart did) or use third-party platforms (Criteo, CitrusAd, PromoteIQ, etc.)? Early on, outsourcing to a vendor was faster. However, as retail media became a core strategy, some large players (especially those with resources like Walmart) opted to build or heavily customize their in-house platforms (often still partnering for certain capabilities). A cautionary tale here is Microsoft’s PromoteIQ: it provided the tech for many retailer ad programs, but by 2024 its largest clients began taking their retail media in-house, leading Microsoft to shutter the PromoteIQ service (Here's who stands to benefit from – or lose to – Amazon's new retail media offering - Digiday). This was a strategic misstep for Microsoft – perhaps not evolving the platform enough or misjudging retailers’ desire for control – and it shows that simply being early wasn’t a guarantee of long-term success. On the other hand, CitrusAd thrived by continually expanding features (including off-site ads and better data integration) and was acquired by Publicis Groupe in 2021 to complement their marketing offerings. Criteo successfully pivoted from third-party cookie retargeting (a business in decline) to “Commerce Media”, positioning itself as a retail media platform for retailers and brands. By 2025, Criteo’s network connects many retailers’ inventory to a broad advertiser base, helping both parties and keeping Criteo relevant. The decision between build vs buy in retail media remains nuanced: some like Target’s Roundel or Kroger Precision Marketing have robust internal teams (often using bits of vendor tech under the hood), whereas many mid-sized retailers still rely on vendors.
Branch – Expansion to Programmatic & Off-Site (2020s): The next evolution: retail media networks extending beyond the retailer’s own properties. Once retailers maxed out how many ads they could show on their websites without harming user experience, growth had to come from new inventory (Retail media’s rise increasingly reliant on offsite programmatic media, report finds | Marketing Dive) (Retail media’s rise increasingly reliant on offsite programmatic media, report finds | Marketing Dive). Thus, we see the rise of off-site retail media: using retailer first-party data (purchase history, intent signals) to target those shoppers with ads on external channels (e.g. across the open web, CTV streaming, social media). This essentially merges with classic programmatic advertising, but powered by retailer data. For example, a brand could use Walmart’s data to target Walmart customers with ads on a third-party site via Walmart’s DSP partnership with The Trade Desk. The Trade Desk, a leading demand-side platform, capitalized on this by inking deals with major retailers (Walmart, Target, others) to ingest their shopper segments. Google and Facebook also enable retail audience targeting in their ad ecosystems via data onboarding (though privacy rules are tightening that). A reported statistic shows how critical off-site has become: offsite programmatic retail media is forecast to reach $20B in 2024, up sharply from $7.5B in 2023 (Retail media’s rise increasingly reliant on offsite programmatic media, report finds | Marketing Dive). Retailers and vendors enabling these off-site extensions are thriving – it’s a major success branch. Criteo, for instance, has built a DSP and acquired IPONWEB to bolster its programmatic capabilities, aiming to serve ads both on retailer sites and off-site exchanges using retailer data. CitrusAd partnered with Epsilon (another Publicis company) to do off-site targeting using loyalty data. Particular Audience is also innovating here: it touts “Open Web Demand Integrations” as part of its roadmap (Particular Audience Announces Largest Ever Product Release—Reinforcing Market Leadership in Advanced AI-Powered Retail Media, Search & Personalization | Business Wire), suggesting its retail media clients will be able to connect with external ad demand seamlessly. This indicates PA’s strategy aligns with the programmatic shift – breaking out of the confines of on-site ads to truly omnichannel retail media (including off-site and even in-store digital signage, potentially).
Privacy and Data Collaboration: Driving this evolution is the push for privacy-first advertising. Retail media leverages consented, first-party shopper data, making it attractive as cookies disappear (Swiftly | Retail Media & Tech Insights | The Evolution of Retail Media). Techniques like clean rooms allow retailers and brands to match data for targeting without exposing PII. We see alliances forming (e.g. retailers joining networks like Amazon’s new Retail Ad Network service, which Amazon launched to provide its ad tech stack to smaller retailers (Here's who stands to benefit from – or lose to – Amazon's new retail media offering - Digiday) (Here's who stands to benefit from – or lose to – Amazon's new retail media offering - Digiday)). Amazon’s move here is notable – after dominating its own retail media, it now offers a hosted solution to others, directly threatening vendors like Criteo and CitrusAd by lowering the barrier for retailers to start (with Amazon’s reputed tech) (Here's who stands to benefit from – or lose to – Amazon's new retail media offering - Digiday). This will be an interesting branch: will retailers choose Amazon’s offering or stick with independent solutions to avoid relying on a competitor? The future will tell.
Outcome: Retail media has evolved from an afterthought to a centerpiece of modern e-commerce strategy. A retailer’s decision to invest in a robust retail media network can mean tens or hundreds of millions in high-margin ad revenue. Successes in this space include Amazon (of course) – essentially creating an entirely new pillar of its business. Also, retailers like Kroger who embraced it early now enjoy strong partnerships with CPG brands eager to spend on their networks. Among tech providers, CitrusAd’s rise and acquisition is a success story, as is Criteo’s reinvention into a retail media enabler for dozens of retailers (turning a looming failure into a renewal). We also saw missteps: Microsoft’s PromoteIQ, which had a promising start, ultimately couldn’t retain clients – it was shut down as clients left for in-house solutions (Here's who stands to benefit from – or lose to – Amazon's new retail media offering - Digiday), showing that failing to continuously differentiate your platform (e.g. with AI or better integration) can cost you even in a hot market. Another potential misstep would be any retailer that waited too long to launch a media network – by the time they do, entrenched players might dominate spend.
Particular Audience’s differentiation in retail media is its deep integration of AI and personalization into retail media. Traditional retail media platforms often focused on ad serving and monetization, sometimes at the expense of the shopper experience (e.g. showing too many irrelevant sponsored products). PA turned this into a tech problem: it uses AI to decide optimal ad placement and timing across search and recommendation touchpoints (Advanced Retail Media Technology), effectively balancing monetization with relevance. This addresses the common “on-site ad overload” issue – with PA’s personalization, every ad is also a relevant recommendation, blurring the line between content and advertisement. Consequently, PA claims retailers can unlock 4-10x more ad revenue versus manual keyword-based ad systems, because AI finds opportunities that humans miss (Advanced Retail Media Technology) (Advanced Retail Media Technology). In the decision tree of retail media, PA chose the path of automation and intelligence over manual ad placement. It also offers a modular, API-driven platform (headless or low-code integration (Advanced Retail Media Technology)), meaning retailers can adopt it quickly – aligning with the “as a Service” branch. All these give PA an edge against incumbents: while CitrusAd or PromoteIQ might require manual setup of campaigns or focus mainly on on-site banners, PA’s fully automated, personalized approach “shifts from manual processes to intelligent automation” (Particular Audience Announces Largest Ever Product Release—Reinforcing Market Leadership in Advanced AI-Powered Retail Media, Search & Personalization | Business Wire), as noted in an industry release. This positions retailers using PA to generate more revenue with less work, a compelling proposition as retail media enters a more competitive, scaled phase.
Strategic Successes and Failures Mapped
The branches above illustrate how strategic choices led some companies to flourish and others to falter. To summarize a few key success vs. failure outcomes:
- Endeca vs. Algolia (On-Premise vs Cloud): Endeca’s failure to modernize (stuck on-prem, no major innovation post-2011) became a cautionary tale (Endeca migration - why now, why Algolia - Algolia Blog | Algolia). Meanwhile, Algolia’s cloud/API-first strategy turned it into a market leader, powering search for thousands of sites by offering what Endeca couldn’t – agility and AI relevance. The decision to embrace cloud early on was pivotal to Algolia’s success and Endeca’s obsolescence (Endeca migration - why now, why Algolia - Algolia Blog | Algolia).
- Rule-Based Vendors vs. AI Personalization Leaders: Early personalization providers that didn’t invest in AI saw diminishing returns. For example, MyBuys (focused on marketing emails and simple recs) eventually merged away, whereas Dynamic Yield’s AI focus led to a $300M acquisition. Those who pivoted to AI (Coveo acquiring Qubit to inject personalization (AI-powered personalization provider Qubit gets acquired by Coveo | VentureBeat), RichRelevance reinventing as Algonomy) stayed relevant. The clear lesson: relying on static rules was a strategic dead-end once data-driven AI proved more effective.
- Microsoft PromoteIQ vs. CitrusAd (Retail Media Strategy): Microsoft’s PromoteIQ enjoyed early success signing big retail clients, but perhaps under Microsoft’s ownership it didn’t innovate enough or remain flexible. The result: clients built their own systems and PromoteIQ was shut down by 2024 (Here's who stands to benefit from – or lose to – Amazon's new retail media offering - Digiday) – a failure to retain market fit. In contrast, CitrusAd continually expanded its offering (self-serve interfaces, off-site ads, first-party data utilization) and gained over half of top 20 global retailers as clients (Retail Media for Retailers - CitrusAd - Sponsored Product Ads). CitrusAd’s acquisition by Publicis validates its success. The strategic difference was staying ahead of retailers’ needs (CitrusAd) versus resting on laurels (PromoteIQ).
- Amazon & Walmart (Retailer Strategy): Amazon’s enormous success in ads prompted Walmart to invest heavily in its own network (Walmart Connect). Walmart’s strategic success was partnering externally (with The Trade Desk for off-site, and acquiring Polymorph for in-house tech) to compete with Amazon’s capabilities (Here's who stands to benefit from – or lose to – Amazon's new retail media offering - Digiday). Traditional retailers who have executed well (Target’s Roundel unit, Kroger’s media arm) are now reaping significant revenues. Those who didn’t (or who partnered with the wrong tech) are scrambling to catch up.
- Particular Audience vs. “Legacy” Approaches: As a newcomer, PA’s bet on unifying search, personalization, and ads via AI is paying off in client wins. It turned potential weaknesses of legacy platforms into its strengths – e.g. where older retail media relied on “outdated keyword targeting” and missed much revenue (Particular Audience Announces Largest Ever Product Release—Reinforcing Market Leadership in Advanced AI-Powered Retail Media, Search & Personalization | Business Wire), PA’s AI prediction captures that upside. Its success is seen in results like higher CTRs and increased ad spend captured (Advanced Retail Media Technology) (Advanced Retail Media Technology). If we consider “legacy” retail media approach a branch, PA effectively leapfrogged it, putting pressure on incumbents to add similar AI capabilities. A failure to note here might be any vendor that tried to offer search or personalization without advertising integration – as retailers and brands now prefer holistic solutions, point solutions risk being sidelined. PA’s rise highlights how a coherent, AI-first strategy can outmaneuver more entrenched players who are slower to integrate or innovate.
In summary, the decision tree of strategy in this domain shows that those who embraced cloud, AI, automation, and integration tend to be the winners. Those who clung to legacy models – whether that’s selling on-prem software, using manual processes, or relying on third-party cookies – often found themselves at a dead end. The market is littered with acquired or defunct companies that took the wrong turn, while the success stories are those that continually chose the forward-looking branch.
Future Directions and Next Innovations
The evolution continues, and new branches are emerging on the decision tree looking forward:
- Generative AI & Conversational Search: The next frontier in e-commerce search is incorporating generative AI (like GPT-4, Bard) to enable natural language dialogues with shoppers. Instead of typing keywords, users can ask complex questions and get personalized answers or product recommendations. Generative AI stands to “revolutionize search and change how we consume information online,” though work remains to improve accuracy (The Evolution of Search: AI’s Impact on eCommerce). Already, we see e-commerce chatbots and voice assistants that can handle queries like “I need a gift for a 5 year old who loves science” and return tailored suggestions. Retailers must decide whether to integrate these AI models (and how to control their outputs). Vendors like Algolia have added features for AI Q&A on top of search, and Particular Audience hinted at “Sentence Embedding” UI to make its AI search tuning easier (Particular Audience Announces Largest Ever Product Release—Reinforcing Market Leadership in Advanced AI-Powered Retail Media, Search & Personalization | Business Wire) – essentially opening the black box of AI so retailers can guide generative or semantic behavior. The strategic choice will be whether to lead or lag in adopting generative AI for commerce. Those who get it right could offer an unmatched conversational shopping experience, while those who wait might appear outdated.
- Omnichannel & Offline Integration: Future personalization will fully break the online/offline barrier. Omnichannel personalization means what a customer does in a physical store will reflect online and vice versa. Loyalty apps, point-of-sale data, and in-store behavior (via sensors or scan data) will feed into the same AI that drives online recommendations. Retail media will also expand in-store (digital shelves, smart cart ads) creating a seamless retail media network across channels. We already see some retailers include in-store data and touchpoints in their media networks (Swiftly | Retail Media & Tech Insights | The Evolution of Retail Media). The winners will be those who successfully unify the customer journey across channels, so that, for example, a shopper gets a personalized offer on their phone as they walk in a store, informed by their online browsing history. This requires robust data platforms and consent management – a complex but rewarding branch to pursue. Particular Audience appears to consider this, discussing omnichannel measurement and even in-store integration via APIs in its materials (Innovative Retail Media Applications of Particular Audience APIs) (e.g. measuring if an online ad led to an in-store sale).
- Privacy-First Data Strategy: As regulations tighten (GDPR, CCPA, and more on the way) and browsers clamp down on tracking, the only sustainable path is a privacy-by-design approach. Future tech will emphasize zero-party data (data customers willingly share) and anonymous personalization (contextual or cohort-based targeting that doesn’t rely on identities). We see innovation in on-device personalization and federated learning, where algorithms personalize experiences without raw data ever leaving the user’s device or the retailer’s secure cloud. Companies that build trust with consumers by being transparent and giving control over data will have an advantage. For retail media, this means investing in clean rooms and secure data collaboration (so brands can match their customer lists with retailer audiences without privacy leaks). It also means solutions like PA’s zero-PII segment builder will become standard (Particular Audience Announces Largest Ever Product Release—Reinforcing Market Leadership in Advanced AI-Powered Retail Media, Search & Personalization | Business Wire) – enabling targeting using rich data signals without exposing personal info. In the decision tree, there is no longer a viable branch that ignores privacy; the choice is between doing the minimum or proactively making privacy a feature. The latter likely wins long-term consumer loyalty.
- Holistic Commerce Media & Network Alliances: The term “commerce media” is emerging to describe the convergence of retail media with all other marketing channels – essentially a future where the distinctions blur and it’s all just commerce-driven advertising. We might see alliances or interoperability among retail media networks (for instance, a brand could book a campaign that runs across multiple retail sites via a unified interface – some DSPs and agencies are working toward this). The Trade Desk and others may facilitate a federated ecosystem where many retailers’ ad inventories can be accessed in one place (with appropriate data safeguards). This could be a new branch: go it alone with a walled garden vs. join a federated network. Amazon’s entry as a service provider to others complicates this too. If smaller retail media networks band together (via companies like Criteo or liveRamp), they can collectively rival the big ones. The strategy here will shape how brands allocate budgets – a more unified buying experience could attract more spend off the duopoly of Google/Facebook.
In all these future directions, companies like Particular Audience are positioned to adapt because they are built on flexible, AI-centric architecture. For example, plugging in a generative AI model or a new data source is easier if your platform is modular and cloud-based. PA’s emphasis on “AI-first strategies” (Particular Audience Announces Largest Ever Product Release—Reinforcing Market Leadership in Advanced AI-Powered Retail Media, Search & Personalization | Business Wire) and its modular API approach (Advanced Retail Media Technology) suggests it can evolve with these trends (be it adding a chatbot interface or integrating a new privacy tech) relatively quickly compared to a patchwork legacy system.
Conclusion: The Decision Tree in Summary
The evolution of e-commerce search, personalization, and retail media can be seen as a series of branching decisions. At each branch – from on-premise to cloud, from rule-based to AI, from manual to programmatic, from siloed to unified – the industry has largely favored the branch that delivers more agility, better user experience, and more revenue. Major vendors have defined themselves by the branches they took:
- Cloud/API Pioneers (Algolia, etc.) vs. Legacy Suites (that faded).
- AI Personalization Leaders vs. Rule-based tools (that were acquired or out-innovated).
- Retail Media Innovators vs. those that failed to scale or adapt.
We’ve seen strategic masterminds (and some blunders) along the way. The companies left standing strong – Algolia, Bloomreach, Coveo, Criteo, The Trade Desk, etc. – are those that continually chose innovation. Particular Audience stands out as a new generation player that from inception aligned to all the winning branches: a unified, AI-driven, cloud platform spanning search, personalization, and media. It serves as a live case study of where the industry is headed: toward convergence of capabilities, powered by AI and first-party data, delivered with speed and flexibility.
In the next phase, embracing generative AI, omnichannel data, and privacy-centric design will be the key branching points. The decision tree is still growing new limbs. Retailers and vendors will once again face choices – and as history has shown, those who anticipate and jump to the next branch early will thrive, while those who cling to the old branch risk getting cut off. The only constant in this journey is the imperative to keep evolving with the customer at the center, using technology as the enabler for ever more personalized and seamless shopping experiences.