In 2017, Andrew Ng (Industry AI leader, Adjunct Stanford Professor, founder of Google Brain and former Chief Scientist at Baidu) said, “Artificial Intelligence is the new electricity. Just as electricity transformed almost everything 100 years ago, today I actually have a hard time thinking of an industry that I don’t think AI will transform in the next several years.”
The technological and financial opportunity AI presents should have been clear to investors - according to IDC, worldwide spending on AI-Centric Systems Will Pass $300 Billion by 2026. With its seemingly limitless applicability, many fields are rapidly embracing Machine Learning and AI, from education, to finance, to healthcare, to ecommerce and so much more.
Oft quoted English science-fiction writer, futurist and inventor, Arthur C. Clarke (most recently by Packy Mckomick of Not Boring) wrote that "any sufficiently advanced technology is indistinguishable from magic". One of the best examples of magic tech in recent memory is undoubtedly generative AI - the ability to create images and articles from short text prompts was the stuff of science fiction a few years ago.
With the release of GPT-3 and Dall-E 2 by Open AI and a flood of exciting rival tech, a sudden wave of optimism reminiscent of 2021 is surging through the VC capital markets as investors pour funding into generative AI. Recent news of multi-million dollar raises for companies such as Jasper and Stability AI reflect the dramatic way this technology has captured the imagination and excitement of not only the general public, but also venture capitalists in the otherwise dreary markets of 2022.
General Partner at Unusual Ventures Sandhya Hegde writes that we are now witnessing “the third wave of Applied AI in SaaS: generative software…scaling unique human-like work output across modalities, including text, image, voice, code, music, 3D models."
While access to generative AI via open APIs is exciting and has already spurred many new ventures, companies built on open APIs accessing models trained on publicly available data (image and text especially) will struggle to remain differentiated.
If data is publicly available then anyone can access it, and over time software models tend to become commoditized as new entrants join the market, meaning that generative AI companies pose significant mid-term risks to investors.
So what are savvy investors looking for in an AI investment in 2023?
Data IP and true data network effects.
What does that look like? Differentiated, proprietary data sets and unique access to scalable, real-time data allows a company to build a long-term competitive moat around its technology.
That’s a lot to break down.
Before we do that, let’s quickly explain AI, Machine Learning, and the role of data.
What is AI and Machine Learning?
AI is the general field of intelligent-seeming algorithms, it involves programming computers to make decisions for themselves, mimicking or simulating human thought or behavior.
A large contingent of AI research today is focused around language and image readability, and generative AI is (understandably) creating a lot of hype.
Machine Learning is a subset of AI that deals with the creation of computer programs that can learn and improve on their own. A class of data-driven algorithms enable software applications to become highly accurate in predicting outcomes without any need for explicit programming.
If you’ve shopped on Amazon or watched something on Netflix, those personalized (product or movie) recommendations are great examples of machine learning in action.
What’s the role of data in AI and Machine Learning?
Data is used to train models in AI and Machine Learning. It’s also used to test and validate these models.
Computer scientist and Co-founder of Y Combinator Paul Graham recently tweeted:
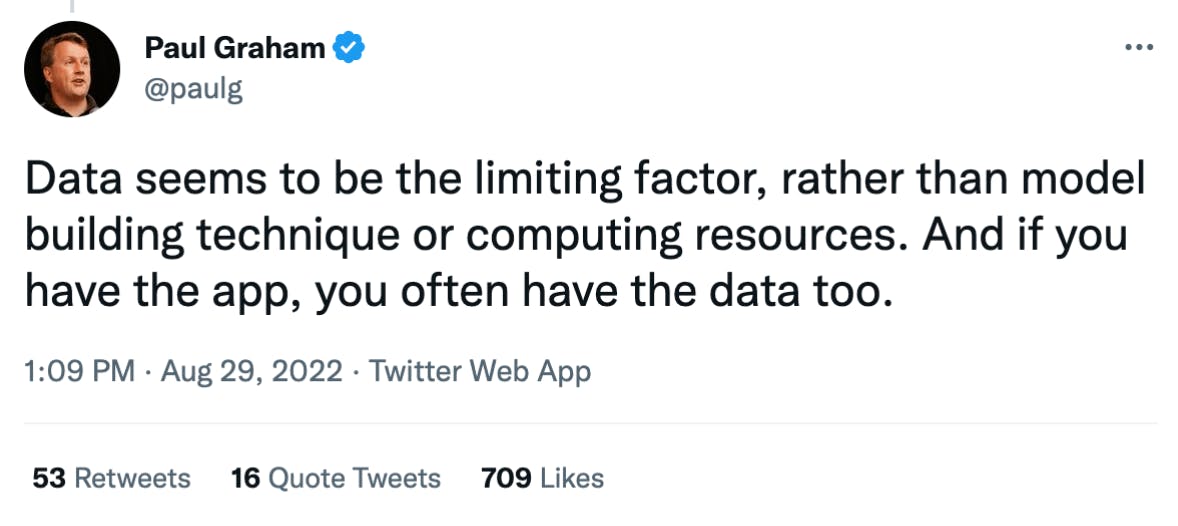
This is something Andrew Ng (more recently founder of Landing AI) also feels passionately about. His campaign for data-centric AI aims to shift the focus of AI practitioners from model/algorithm development to the quality and scale of data they use to train models. Andrew believes that data is central to the future of AI and Machine Learning - not just model development. In fact, models in many applications are a solved problem, with most having been written as long ago as the 70s.
Making ML models more performant in real time computational environments requires bigger, cleaner, better and stronger datasets - but if those data sets are public and available, the models, while performant, will be commoditized.
In order to create genuine differentiation, a company must further develop their models through proprietary data.
What is proprietary data, and why is it important?
Proprietary data is data that is owned by a specific company or individual. This data may be confidential and may not be shared with others without permission from the owner.
- It allows companies to train their algorithms on a more diverse dataset, which in turn leads to more unique results.
- It gives companies a competitive advantage over other companies that do not have access to the same data.
- It allows companies to create customized models that are specific to their own business needs.
Partner at Canaan and respected deep tech investor Rayfe Gaspar-Asaoka believes that:
“To deliver on the promise of disruptive change, AI must create differentiation… There is a strong feedback loop between AI algorithms and data. The better the data, the better the algorithm will perform at future predictions. And the better those predictions, the better the output data, which is then fed back into the algorithm…a company with even the slightest head start with a better proprietary data set or algorithm will have an ever-increasing advantage over their competitors. This winner-take-all characteristic of AI is one of the things that makes these companies so powerful.”
Martin Casado of A16z famously wrote about the (empty) promise of data moats - it can be easy for a company, platform or product to generate data and claim they have a moat of some form, however this is often incorrect. He concludes that for data IP to be defensible and scalable, it has to be scant, unique, rare, high quality and difficult for others to obtain - ergo ~ideally, proprietary.
The Web is not proprietary, is it?
Generative AI in its current form trains on large bodies of publicly available data, and as models become a solved problem, they risk becoming commoditized.
Unique data becomes the defining factor for the future of a platform or product. This is nothing new.
We know from other domains, especially within the walled gardens of big tech, that collaborative (wisdom of the crowd) data used to help link entities is often what makes those platforms so uniquely intuitive, engaging and sticky. Over and above what they might achieve by only understanding the relationship and shared contexts of textual and image metadata.
Take information retrieval. In theory, (given sufficient capital and smarts) anyone can crawl and scrape the web using Natural Language Processing and Computer Vision to develop an understanding of the web, via the information it is made up of. In fact, anyone could even proxy relevance or popularity by mentions or backlinks (*ahem*, Google!).
The proverbial gold mine(s) on the web depend on proprietary collaborative data.
Consider your TikTok feed, or your Netflix interface for example. It’s not just your behavior, but the similarity of that behavior to the journeys of other users that informs your 'magical' (intuitive, personal, automatic) scrolling experience.
In fact, collaborative data is so effective because users are clever enough to navigate shortfalls in metadata, reducing dependency on machine readability to filter relevant information.
What is the importance of data network effects, and how does proprietary data add strength to them?
Most applications have a data scale effect at best.
A data network effect is a situation in which a product or service becomes more valuable as more people use it. This is because the more people who use the product or service, the more data is generated, which can be used to improve the product or service.
For data network effects to truly exist, data needs to be real time and should decay quickly (otherwise it may be easier to replicate or copy!).
An excellent example of this is Waze, the community-driven navigation app acquired by Google in 2013.
Waze uses real-time data from the app's users to provide the best route to the user's destination, taking into account accidents, traffic jams, speed traps, construction, and other obstacles that could slow down a driver. Updates are submitted automatically as users drive and can also be proactively shared via the app.
Network Effects Advisor, investor and author of Breadcrumb.vc Sameer Singh believes that the effectiveness of a network effect can be measured by how fresh data is. If data has a fast decay rate, that actually makes it more defensible.
Let’s take Waze as an example again. Real time information about traffic and road conditions is only useful to a user live as they drive and is ‘fresh’ for minutes to hours at best - information about a traffic jam on Thursday simply isn’t useful by Friday.
Another example, where our Waze example is focused on the physical world, would be Similar Inc which does something similar for the online world.
Web crawling aside, the anonymized collaborative data of a community of users contributing real time, quick decay data to benefit the other users of the browser extension creates data network effects - doing for prices, availability, trends and intelligent online navigation what Waze does for navigation in the real world. Both filter relevant information to future users.
For reasons such as these, applications that use collaborative, proprietary data and are able to achieve powerful data network effects are both defensible, scalable and likely to remain differentiated for much longer.
So what should investors know about applied AI in Software as a Service?
The intersection of AI and SaaS is particularly exciting as founders tackling previously unscalable bottleneck problems are outpacing legacy competitors.
Infrastructure and compute simply didn’t exist a few years ago as it does today.
Notably, the playbook for scaling SaaS businesses in the AI era is changing, and so too are the ways investors value and fund innovative companies at the cross section of these models.
What do venture capitalists expect of a SaaS investment?
An abbreviation for ‘Software as a Service’, SaaS providers offer applications to customers via the web. As of 2022, the SaaS market has a value of $186.6 billion, and this is projected to to grow to $700 billion by 2030.
SaaS is a popular delivery model for many business applications, such as CRM and office productivity software.
Venture Capitalists have a tried and true playbook for investing in SaaS companies based on predictable, recurring revenue that can be grown by acquiring more subscriptions at little additional cost.
VCs look for a few key things when considering a SaaS investment. These include:
- A large addressable market.
- A defensible and scalable business model.
- A clear Ideal Customer Profile (ICP).
- A high degree of product/market fit - a sticky product characterized by high usage and low churn.
- An efficient and targeted go-to-market (GTM) strategy.
- Shorter sales cycles – since you have clear focus on a repeatable pain point and value proposition.
- Highly focused product roadmap driven by exact customer pain points.
- Efficient growth managing deal size vs. customer acquisition cost (CAC).
- A path to profitability with high margins from early in a company’s lifecycle.
What investors typically face with traditional SaaS businesses is a period of rapid high growth that plateaus over time. This curve doesn't necessarily flatten, but it becomes harder for the business to grow at the same pace - meaning that when you invest in this type of company, the multiple at which it is valued generally decreases as the growth rate starts to plateau*.
*Before you start acquiring other businesses and growing inorganically, of course.
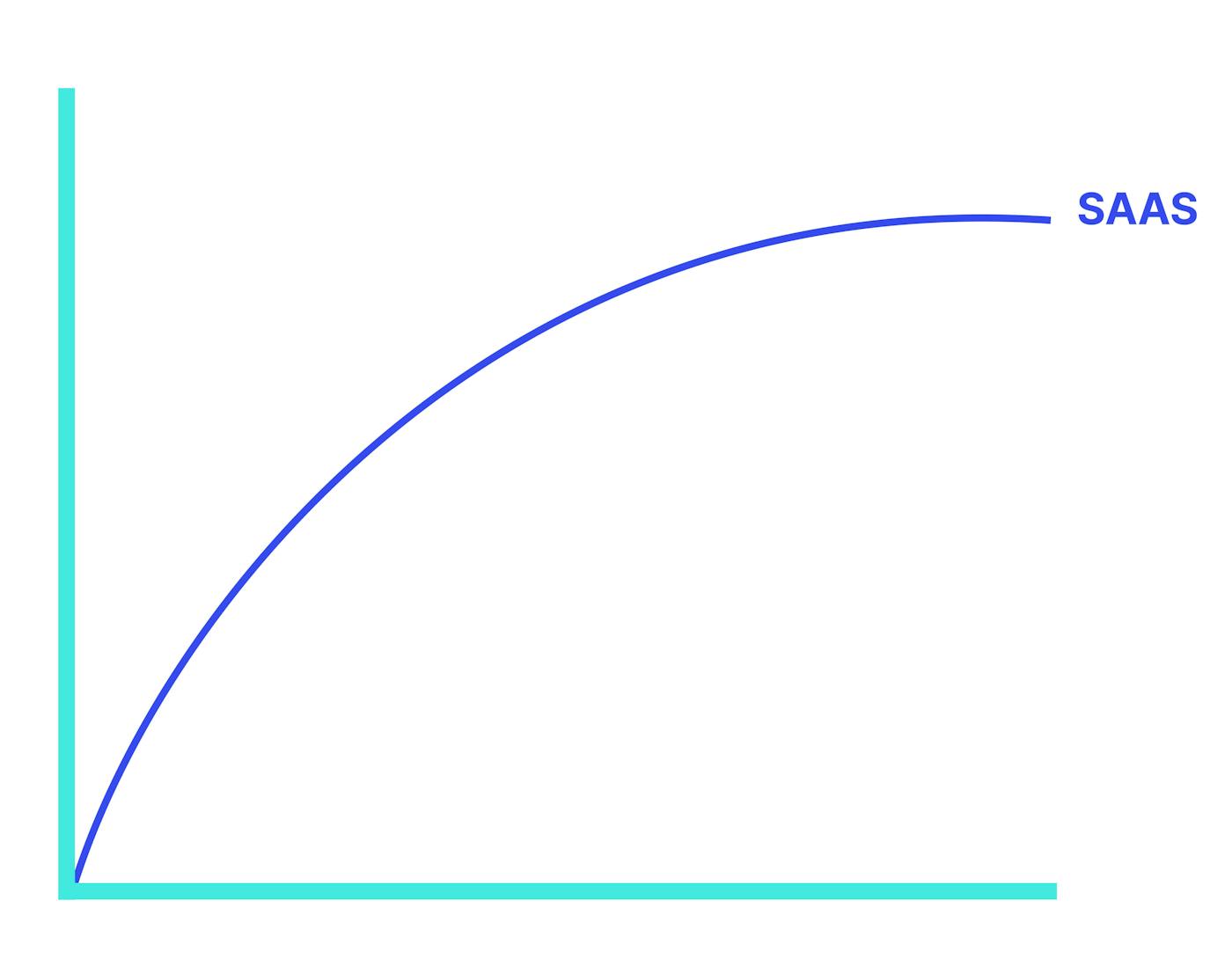
How does this compare to AI startups?
AI startups are those that utilize highly specialized technology. They typically require a high level of investment in research and development (RD) in order to create their products and have much higher up front costs from an infrastructure perspective.
What do venture capitalists expect of an AI startup?
Investing in AI startups can be a riskier proposition than investing in other types of startups, but it can also offer a higher return. In an article published in 2020, Forbes estimated that:
“AI companies require 3-6x greater upfront investment as compared to traditional SaaS companies but in return have 3-6x bigger market opportunity.”
Large scale data processing and fixed cost infrastructure makes margins negative from day 1, and lower than traditional SaaS until scale is reached.
For example, a Series A stage HR management software SaaS company might spend $10,000 a month on cloud costs, while an AI Machine Learning company might spend as much as $100,000.
Depending on the underlying infrastructure of data pipelines and GPU compute capacity, as the scale of data used in processing, cleaning, reporting and training models grows so does cost.
AI companies experience growth in a slightly different way to traditional SaaS, although can often exhibit similar monetization paths from early applications of their product or platform. Other sorts of progress unique to AI SaaS companies include huge leaps forward related to technical breakthroughs, innovations, optimizing compute, reduction in cost of delivering technology, in addition to commercial successes such as increased user numbers, use cases or revenue.
If you were to chart this, you’d see flatter periods of growth followed by steep jumps in progress.
Nevertheless, higher upfront investment in AI companies can pay off at scale, given significantly more applications for a platform or technology.
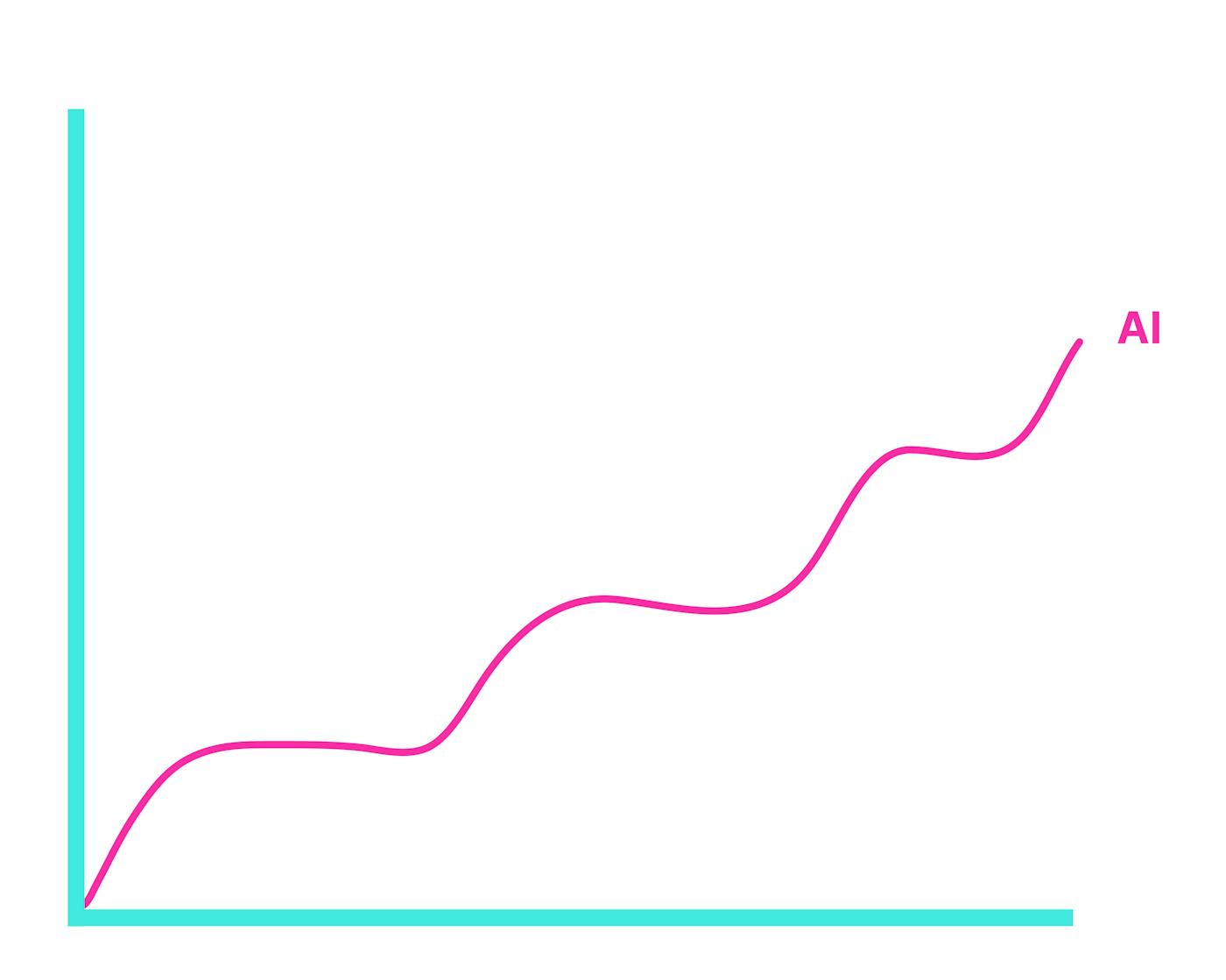
Hybrid SaaS and AI business models stand to strengthen investment candidates and unlock hyper growth
An AI SaaS company is often more defensible vs traditional SaaS thanks to deeper technical moats and in the best cases unique proprietary data ranging from data scale effects to the elusive data network effect.
These businesses can benefit from economies of scale that come with being a hybrid model. They're attractive to investors because they often have a lower risk profile than pure-play deep tech AI ventures, and can offer a unique combination of software and services that are both commercially lucrative and difficult for competitors to replicate.
Relative to deep tech AI, hybrid AI Saas companies enjoy higher margins, high growth, low churn, and high net revenue retention, but they also present the opportunity for a future ‘hockey stick’ commercial outcome. A hybrid AI Saas company can unlock hyper growth given proprietary data that compounds Gaspar-Asaoka’s “...strong feedback loop between AI algorithms and data. The better the data, the better the algorithm will perform at future predictions”, enabling more applications of a platform, through both adjacent markets and new commercial outcomes within the algorithm/data flywheel.
Additionally, SaaS brings with it a number of advantages that can help a hybrid AI startup scale revenue early and efficiently when compared to traditional deep tech companies.
For example, recurring (or repeat) revenue models give startups a more predictable cash flow, which is helpful for capital intensive ventures that involve building and sustaining robust AI infrastructure.
Moreover, subscription and usage-based pricing models give startups the ability to price their product according to either the value it provides or cost-plus-margin; whichever suits a target market and business model best. This is a particularly important consideration for AI startups, as the cost of developing and acquiring the datasets needed to train their algorithms can be high too.
Finally, the SaaS business model gives startups the ability to reach a global market quickly and efficiently. With the help of the internet, SaaS companies can sell their products to customers anywhere in the world, without the need to establish a physical presence in each market.
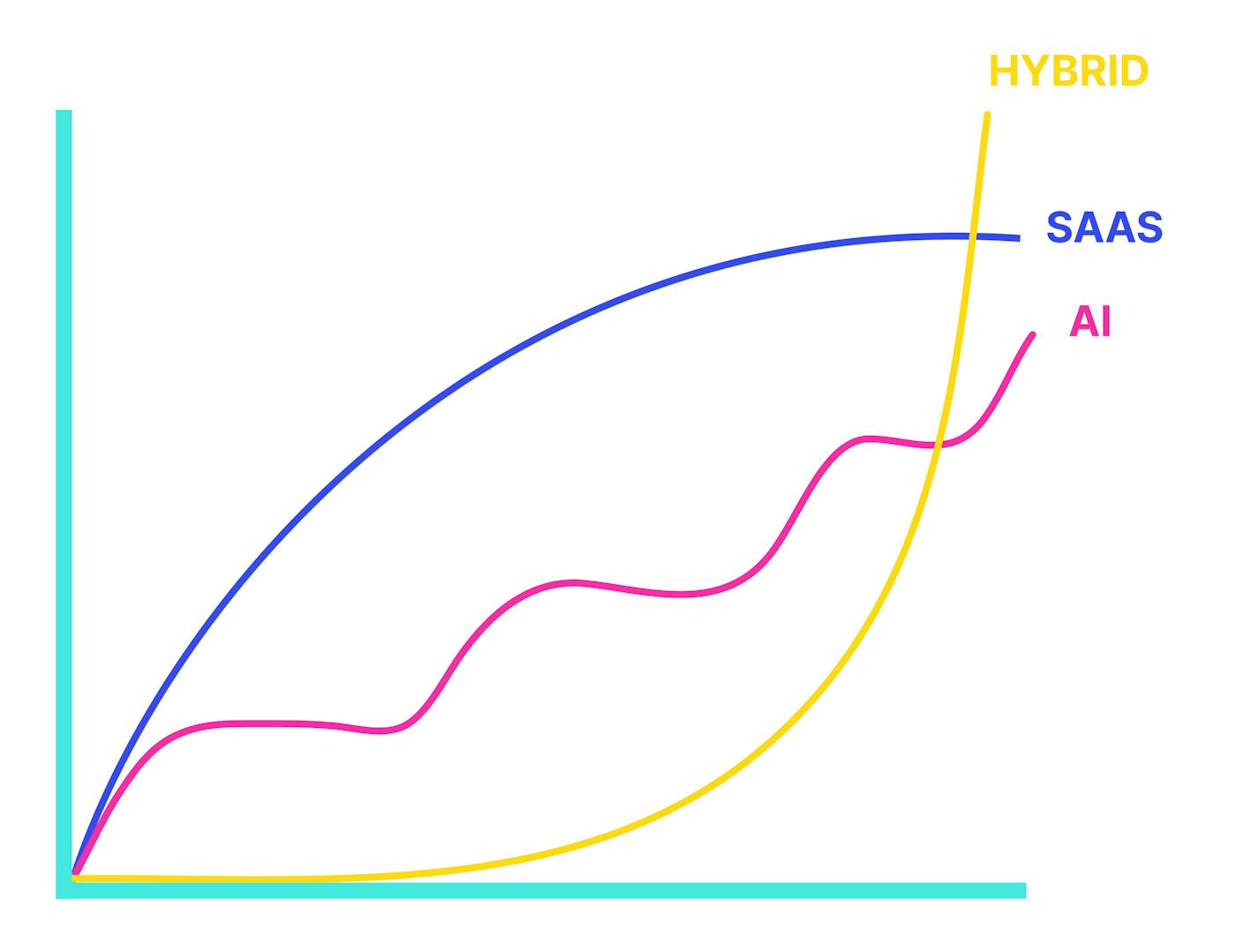
The challenge for investors lies in not pigeonholing an AI SaaS company as its first SaaS application, but in understanding all tangible future potential. Often that first commercialization may be just one offering within a future suite of products a company provides while world changing technology is developed. Fortunately, the infrastructure, expertise, and IP generated in the short term is often applicable to long term product and commercial outcomes.
In short
Every investor wants to get in on the ground floor of new and exciting technologies, but depending on what level of risk they’re willing to stomach, a pure AI startup might be deemed too speculative. However, a startup that combines AI research and development, data acquisition and infrastructure investment with another, more established business model such as SaaS can make for a more compelling investment opportunity.
For those looking to invest in hybrid AI startups, the potential rewards are significant, and when it comes to investing in AI, savvy investors know that true data IP and data network effects based on proprietary (typically collaborative) data are the most defensible asset a data startup has.
Does a company have a solid data strategy and an objective use case, and is the quality of that data both scalable and defensible?
The right data is even more important than the algorithms, and without it, a company will have a difficult time developing a competitive advantage.